S4-Episode 4: Artificial intelligence in health care: Balancing innovation with privacy
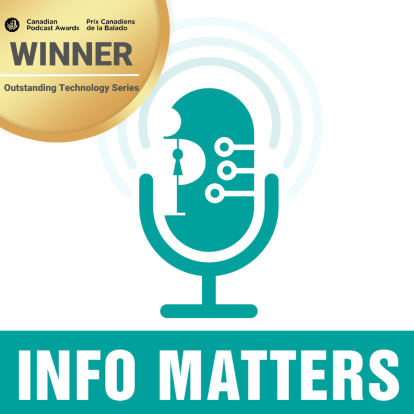
Artificial intelligence (AI) has the potential to accelerate and improve many aspects of health care from diagnostics to treatment. However, the use of AI in health care also raises significant questions about privacy, patient safety, ethics, and transparency. Dr. Devin Singh of Toronto’s Hospital for Sick Children speaks about balancing the benefits and risks of this transformative technology.
Notes
Dr. Devin Singh is an emergency physician and lead of clinical AI and machine learning in Paediatric Emergency Medicine at The Hospital for Sick Children (SickKids). He is also co-founder and CEO of Hero AI.
- Combining medicine and machine learning [2:21]
- A patient death as a spark for thinking differently about health care delivery [3:27]
- Demystifying the integration of AI into pediatric emergency medicine [5:03]
- Real world applications of AI at SickKids [7:31]
- Privacy and ethical considerations when implementing AI [9:04]
- Mitigating bias in data, ensuring representative data models [11:26]
- Transparency and obtaining informed consent from patients [14:05]
- Human oversight of AI systems and predictions [16:05]
- Respecting patient autonomy and decision making [20:10]
- Guarding against cybersecurity risks [23:02]
- How doctors use AI scribe technology [25:34]
- Predictions about the future of AI in health care [29:16]
Resources:
- AI scribe (OntarioMD pilot project)
- Privacy and humanity on the brink (blog by Commissioner Kosseim)
- IPC Strategic Priorities 2021-2025
- Trust in Digital Health (IPC resources)
Info Matters is a podcast about people, privacy, and access to information hosted by Patricia Kosseim, Information and Privacy Commissioner of Ontario. We dive into conversations with people from all walks of life and hear stories about the access and privacy issues that matter most to them.
If you enjoyed the podcast, leave us a rating or a review.
Have an access to information or privacy topic you want to learn more about? Interested in being a guest on the show? Send us a tweet @IPCinfoprivacy or email us at @email.
Transcripts
Patricia Kosseim:
Hello, I'm Patricia Kosseim, Ontario's information and privacy commissioner, and you're listening to Info Matters, a podcast about people, privacy and access to information. We dive into conversations with people from all walks of life, and hear real stories about the access and privacy issues that matter most to them. Hello, listeners, and welcome to another episode of Info Matters.
Artificial intelligence is on everyone's minds these days, dominating headlines around the world. AI offers tremendous opportunities in many aspects of our lives with its real-world benefits unfolding in real time. For Canada's overburdened healthcare system still recovering from the effects of the pandemic and serious shortages of healthcare workers, a little help from AI could be truly transformative.
Integrating AI tools in healthcare could help alleviate administrative burdens, enhance resource management, and improve the overall patient and staff experience. This all sounds good in theory, but the integration of AI into healthcare raises significant questions about privacy, ethics and transparency. How do we know that sensitive health data will be protected?
Is the data being used to train AI algorithms biased? Can we trust technology alone to make the right diagnoses? Our guest today is going to help us unpack some of these complex questions. Dr. Devin Singh is an emergency physician and lead for clinical AI in pediatric emergency medicine at Toronto's Hospital for Sick Children.
He's also the co-founder and CEO of Hero AI, a company dedicated to leveraging AI to solve some of healthcare's greatest challenges. Dr. Singh, welcome to the show.
Dr. Devin Singh:
Thanks, Patricia. I'm really excited to dive into a lot of the topics you just raised. There's so much to talk about, so I can't wait to get started.
PK:
Absolutely, there's lots to unpack. Before we start into the heart of the matter, maybe you can first tell us about your journey in medicine and technology.
As a specialist in pediatric emergency medicine, what inspired you to pursue clinical AI and machine learning at Sick Kids?
DS:
I didn't have a technical computer science background before going into medical school. I was always into technology, always into computers and the latest things, but certainly I wasn't formally trained in that. I actually ended up doing my medical school training at Sydney University Medical School out in Australia.
And was really blessed to have an opportunity to come back to Toronto and do my pediatric residency training at the Hospital for Sick Children at the University of Toronto. It was actually throughout my training, that you start to get exposed to the realities of healthcare. When you're a medical student, sometimes you look at these large, ivory tower organizations and the prestige that comes with it.
And you don't realize that there's some harsh realities around healthcare that you just don't see until you're there and you're working. Some of those harsh realities involve themes that you brought up in your introduction around patient wait times, surges in patient volumes. The demand sometimes outstripping the resourcing that you have on the ground, even in the best institutions in the world.
I started to think through how do we rethink healthcare delivery on a system level? There's a particular case that came through of a patient who really, sadly died. What possibly could have been a preventable death if our health system was designed in a different way. I remember being a junior member on the team and doing CPR on this kiddo, and quite literally feeling them slip away beneath my hands.
When you see the impact on a little kid and you do the case review and think, "If these few things were different, I genuinely feel like that kid could be alive." That is really powerful and struck a chord with me, and lit a fire that led me to start thinking differently. I started to think about quality improvement and patient flow, and I'd become expert in that.
I started to realize that these things wouldn't scale really well. These things alone weren't going to do it. I accidentally stumbled across machine learning and AI and had some really great mentorship around it. Suddenly, a light bulb went off and a lot of the dots between the different facets of the problem that went into this terrible case, I realized could possibly be solved by AI.
Then I became obsessed. I taught myself how to program and write machine learning code, and then was able to get into the University of Toronto to do my master's in computer science. They really mentored me technically, theoretically, practically on how to understand applications of machine learning into healthcare. That's where I'm at right now.
PK:
That is a tragic story, but also a powerful example of how real life can really inspire a career trajectory, that in your case, is really transforming healthcare at Sick Kids.
Now, for a lot of listeners, it may be helpful if you could demystify some of your work. What does your typical day look like? What are some of the more basic use cases for integrating AI into pediatric emergency medicine?
DS:
My work is really diverse in the sense that I still work clinically as an emergency doctor in the hospital. Then I have a portion of my time that is protected and dedicated to thinking through these applications of AI and machine learning and healthcare. Looking at a specific problem like the one I described, and thinking about how could we leverage this method of machine learning to solve for it?
So let me give you a tangible example. In this case that I described, what if we could use a machine learning model to actually predict what the downstream tests might be for kids who present with common conditions like appendicitis, urinary tract infections, maybe broken arms? If we could use a bit of data when someone initially arrives to the emergency department and predict that they're going to need that test.
Well, could we potentially have an AI system just order the tests right when you arrive? Maybe you don't need to wait six to eight hours for a doctor to tell you the obvious to say, "Yeah, let's get an ultrasound." Then you wait another couple hours to get the ultrasound done and then you get your diagnosis. What if we could actually train a machine learning algorithm to make that prediction?
Then just automate ordering it so patients can get to the diagnosis faster, get to treatment faster. This is using AI as a method to solve a real-world problem and improve care. When we talk about demystifying it, I think it's important that the audience realizes that majority of the machine learning models we build are these custom models in-house using patient data.
Then making predictions that are then safe, ethical, responsible, and are the things that patients are actually asking us to do, so it involves co-design with patients. Most of my job involves doing that, is trying to take what I see in my clinical practice in providing care. Then trying to find the problems and solve them in the way that I hope patients want them to be solved.
PK:
That's a good example of a use case that accelerates the required medical tests that need to be done, in order for eventually the physician to meet the patient, diagnose the issue and get on with addressing it through appropriate treatment.
Are there other examples that you can share with us that have real-world implications or applications at Sick Kids today?
DS:
We've got ones that are using imaging data, for example, to help get medical imaging faster, get to the right diagnosis faster, get to the treatment faster, and allows us to be a lot more precise in the way we deliver therapies. We've got use cases around thinking through even small molecules and biomedical markers, and genetic markers in patients.
Can we actually take diseases and understand their molecular and their genetic causes that contribute to the disease, and build targeted therapeutics that quite literally helps to undo a disease process? There's one around predicting cardiac arrest in our ICU, where we have real-time data coming into a machine learning model.
We're investigating can we use a machine learning model to predict that this patient may have a cardiac arrest and mobilize a team to that bed ahead of time, which gives us a heads-up? Even if it's just a few minutes, that difference can be lifesaving for patients.
PK:
These are all great examples in the microcosm of Sick Kids. But you could imagine when amplified throughout the healthcare system, how many powerful solutions lie out there in order to help alleviate the burdens on what is an overburdened healthcare system.
This all sounds very exciting and very promising, but as you and I know, there are some downstream risks of using AI. What are some of the privacy and ethical considerations you encounter when implementing these exciting AI applications in healthcare?
Particularly, in an institution dealing with young children, how do you address these concerns?
DS:
During this journey, particularly over the last five years when we were building out these technologies, we recognized that there needed to be governance, rigor and thoughtfulness on a few particular themes. Things around privacy, the regulatory landscape and ethics. And thinking through how do we actually navigate that landscape internally at Sick Kids, which is one thing?
But how do we take these technologies that we're building and actually scale them across the country? That's really the mission, to truly make a global impact on the way we transform pediatric care. In order to do that, it's actually not necessarily the technology that's the hardest part. It's thinking through the risks around privacy, around ethics, around patient safety and governance.
From a practical perspective, we spent about four years designing something called I Get AI Framework, that really ensures the way we build and develop technologies internally, goes through the necessary checks and balances at every single stage from day one. None of these projects get off the ground without thorough regulatory assessment, which considers things like impact on privacy, impact on patient safety.
And an assessment around what are some of the potential, unintended consequences that may happen to the system as we start to move the technologies forward?
PK:
Do you have some examples of what are some of the unintended consequences that you've guarded against at the front end of some of these technologies?
DS:
A great example is bias. We know that the data in our health systems will just naturally have a bias in it. That's human nature. That's what's encoded into the data. When we are training machine learning algorithms, we really think through bias. How are we going to then prove to ourselves that we've done a thorough bias assessment before we deploy a machine learning algorithm?
If you weren't to look at that, you might accidentally deploy a tool that at scale is starting to cause harm to a particular population. The thing is, these are our vulnerable populations potentially that may be at risk, that aren't necessarily well represented in the data. There's things that we do to combat that. First is to really make sure that the way you are assessing bias is incredibly rigorous.
Because the most dangerous thing is to think you've done it but you actually haven't done it properly. There's a few different methods. You could have a technical solution around how you encode the machine learning model to understand the data that can reduce bias. But then you could also say, "Wait a minute. Why is it that if you speak a particular language, you have a bit of a difference in your care pathway?"
And you actually then go back to the clinical space. It's actually going back to where the data is generated in the clinic and saying, "What's happening here? Did you guys realize that there is this bias here?" When you do this rigorous assessment early on, it allows you to create changes in the clinical environment. Another way to tackle this is to bring our data together.
Especially when it comes to pediatrics where we don't necessarily have this massive volume of data in any particular site, you actually need centers from across the country to come together and to solve these problems together in a unified way. The technique for mitigating against bias is making sure we have all the people of Canada represented well in the data that we train the models.
It brings up really interesting questions around how do you navigate privacy in that situation? Is it okay that someone could take data from a whole bunch of different provinces, and then physically move it to one province? Or maybe that's not a good idea. Maybe should we actually think through types of machine learning techniques where you keep the data in the different provinces, and train a bunch of smaller models and then pull the models together?
How do we navigate that so that we can leverage data for everyone, so that they're represented in these models so we can mitigate against that bias? This is where the intersection between the technology's performance, privacy and bias, and safety occurs.
PK:
That's a great example and a great discussion of how you address bias. How about some other aspects?
Like how do you address questions of consent and transparency with your patient population and/or their parents, who are substitute decision makers in many cases?
DS:
Transparency means a few different things. It means making sure that a patient is aware when decisions about their care is being influenced by artificial intelligence. I think that patients want to know that. We are incredibly transparent to our families and to our kids around what technologies are being used and when. It's also very important around having those communications facing the parent or the decision maker.
But also having them developmentally appropriate for that kiddo as well, because the kids want to know. They're so engaged in this conversation. We actually have something called the AI Youth Council, where as we build these projects, we are actively co-designing with our youth to make sure that their voices and opinions are in it. I'm telling you, these kids are really, really savvy. They're talking about privacy, cybersecurity.
They don't want to get accidentally poked for blood work because an AI said they should but they didn't need to. But then another thing is around consent. Informed consent involves that person truly understanding what is the model actually doing? In order for a clinician to then gain informed consent, they need to get an understanding of what the technology is doing as well, so that they can communicate that to the family.
There's a whole piece around AI literacy in healthcare so that the clinicians using the tools understand what's going on, and have frameworks to be able to get that informed consent, making sure that parents and children have choice. That it's okay if in this particular situation, you prefer not to have artificial intelligence augment your care. That should be okay.
PK:
Very interesting, and I agree about the savviness of young people today on many of these issues. We ourselves have a youth advisory council at the IPC, who advise us on questions of privacy and transparency among children and youth, and how to have these conversations in meaningful ways.
And how to adapt them in age-appropriate ways that are matched with the level of understanding of children that we should never underestimate, for sure. Another question I have is, and you alluded to it, how do you ensure that if the AI model is predicting a certain clinical outcome and recommending a medical test?
How do you make sure that that's actually correct and accurate, so that people aren't undergoing medical exams that they don't need to, particularly if there are risks involved? What level of a human oversight is there over these AI models?
DS:
It really depends on each of the individual models and how they will be used in practice. So let me give you an example, because we talked about that idea of using emergency department triage data to predict the test for someone, let's say appendicitis. So predict that a patient needs an abdominal ultrasound, and then just get the test ordered automatically.
Well, in that scenario, we then need to look at is the model actually performing well, which means how many times it gets it right? We take the model and we build it using old data. Then if the model looks like it's working well, we'll plug it into a real-time workflow. Which means in real time as patients are coming to the emerge, the model's making a prediction.
But we don't show anyone the results yet, because we're just still validating is the model really working as we expected in the real-world environment? Then when we obtain strong performance in that scenario, it then becomes, "Well, if we were to actually turn it on and the model gets it right, what happens?" Well, that's easy because we want to accelerate the care, as you said.
But then if the model says you don't have appendicitis, in this case, we are not going to just send you home blindly. You'll go through the normal standard of care pathway, see a physician, and if you needed that test, you'd get picked up then just like you normally would. Then we would mitigate the risk against missing that. Let's take the same model now and move it into a patient's home.
What if we're having patients use a similar type of model that puts their data into an app? The app will say, "Yes, you might have appendicitis, go straight to diagnostic imaging." Wow, you would get to a diagnosis that much faster. But what if the model gets it wrong and it said you don't, but you actually did? Well, now you're in the home.
What's the safety net there? What's the oversight there, and the human workflow there that mitigates against that risk? I give these contrasting examples, because it just highlights the point that it's actually less about the model and the machine learning itself. It's more about how you intend to use that model, understanding then the risks of when the model gets it wrong.
Along this journey, you co-design this with patients and families so that they understand that, "Okay, here's what you're anticipating. Is this the workflow that they actually want?" That's really important, because you can make a lot of assumptions as a leader in AI and in the clinical practice, but those assumptions can often be wrong. You really need to co-engage with families and patients.
Build out these novel workflows with them hand in hand, so that when you do deploy it, you get it right. The last piece around oversight is once the model is deployed, it's not done. You have to make sure that the performance of the model is maintained over time. There is an aspect of human oversight that needs to monitor that and make sure that safety is maintained.
PK:
Another issue sometimes I worry about and doesn't get a lot of play in some of these discussions. How do you factor in the right of people not to know certain diagnoses that may be life-changing? It's one thing to get to those diagnoses early, so that people can take actionable steps or have the choice of taking actionable steps to help alleviate or mitigate those clinical risks.
But what if the situation or the diagnosis is of a clinical outcome that is not actionable? That patients really can't do anything about and can be life-changing, in terms of informing that individual of say, a shortened lifespan or a debilitating disease that has no possible remedy.
How do you respect the right of some people not to want to know that, and to want to live their life fully and enriched by the prospect and potential of the full life experience that's ahead of them?
DS:
Really, what we're getting at there is how do we ensure we respect patient autonomy and patient decision-making? If you're going to do that in a genuine, authentic way, you need then transparency around a process. One example could be that you are part of a family practice that has an electronic health record system.
And that system then uses a whole bunch of different AI models looking at your data to make predictions about maybe future disease states. Maybe one of those predictions is exactly what you said, is something that is life-altering, that is something you did not want to know.
I think that to maintain patient autonomy and to respect that, before that prediction is even necessarily made, does that clinic say, "Here are the suite of things that we could actually run on your data as you interact with us"? Here's what it means to actually get a prediction of these kinds and why it could be valuable.
Here are the areas where we're not so sure. It's a heads-up notice, do you want to know about these things, yes or no? We're going to have to think through how we build in these processes to simultaneously allow for the value of artificial intelligence to truly transform healthcare, but that simultaneously respects patient autonomy, and control and privacy.
Someone may want their data not to be used in this one instance, but they still want it to be used for these other 10 things. How do we facilitate that process, is going to be interesting to see how it plays out.
PK:
One of the things you mentioned in your earlier use cases, is around applications using local data, local machine learning models, locally designed and co-designed with your patient population.
When you say local, how local really are these technologies? How secure are these technologies in terms of, say, their interface with commercial vendors, or their vulnerability to bad actors and cybersecurity risks?
DS:
When we start to think through answering that question, there's so many different facets to it. The first one is cybersecurity and data governance. We really obsess about what are the highest bars of security that then enables privacy to be put into place? For example, when data is in transit between where it lives in a database and to an application or to a model.
And then when data is stored subsequently after that, it is always encrypted. We have to make an assumption that one day there could be a hack. If there was a hack, everything has to be encrypted so no sensitive patient data ever is compromised. It's just a minimum bar standard, but it doesn't necessarily mean every single player in the space does this.
I think it's okay for patients in the community and other practitioners, even family clinics, family practice clinics that are looking to adopt technologies and are looking to vendors around, "Could I use this AI tool or a digital scribe, for example, can I use this tool safely and is it going to be secure?" I would say to the community, challenge those vendors with questions around what happens if the data is hacked?
Tell me all the elements that aren't encrypted. Why aren't they encrypted? How do you control who looks at my data? Do you ever use data for anything other than what we are contracting for and why? What is it that you're using it for? Can I disagree on behalf of all my patients that we don't consent to you using data in that way?
As a health community, we need to challenge and ask those questions so it's crystal clear the measures that are put into place. Then we need to hold our technology companies, and that may be holding our hospitals as well, to these really high standards of maintaining cybersecurity, encryption, data privacy, governance and ethics.
PK:
You mentioned digital scribe and the Ontario government, as you know, recently announced an AI scribe pilot project aimed at helping physicians spend less time on paperwork and more time with their patients. In simple terms, what does AI scribe do?
How game-changing is it? How confident can Ontarians be that some of the checks and balances you've just discussed, are embedded into this pilot project as it rolls out?
DS:
What is the digital scribe? Well, imagine you are a patient and you're sitting in the room waiting for your doctor to walk in. Your physician walks in, starts talking to you and asking why you're there, but is sitting at their computer typing away. That's what happens now. It's an example of how technology and electronic health records has undermined the human-patient connection.
A digital scribe deployed in that office ends up being this different workflow. Patient is sitting there waiting for the physician to come in. Physician walks in, sits down and looks directly at you and doesn't touch their computer at all. Because what the scribe is going to do, it's going to hear that conversation, that audio. It's going to convert it to text.
It's then going to take the text and convert it into that physician note and draft it up for that physician automatically. So that the physician can sit and focus more on that human connection with the patient. Ask a bit more questions about the history, listen to a few extra problems that you're experiencing.
And have a much more human and robust, higher quality interaction with a patient, so that we get to improving patient safety and better patient outcomes. But it's simultaneously addressing the biggest pain point that is contributing to burnout in our physicians, which is this massive administrative burden, where most physicians spend 60% of their time sitting at the computer writing their notes.
That's not what those clinicians signed up for when they went into practice. They signed up because they really wanted to help people. Digital scribes brings this promise, but to your point, there is a lot of risk that needs to be mitigated. The thing is the risk can be mitigated and the promise of this technology, I think, can be realized and will be game-changing.
You have organizations like the OMA, for example, that are doing these evaluations, one on the efficacy of the scribes. But two, on helping these clinics understand what are the types of scribes that you should consider using and what are the ones that are no-go? For example, if all that data that's stored in a transcript was then secondarily sold to another company to then mine your interactions to create profit in some other product, that's probably a no-go.
Whereas if you've got a digital scribe where the data is only stored temporarily, is used for your interaction, is stored in Canada, is always encrypted, is deleted after the fact when the need is gone. A much more rigorous and safe approach to leveraging the data, but making the data work for the patient, not for other people. Then that's a scribe that maybe you would want to use.
The OMA, I was really excited to hear that they are doing a rigorous evaluation, and coming up with a guidance document to help family practice think through the utilization of these types of technologies in a very safe, privacy preserving, highly-regulated way.
PK:
Last summer or a while back anyway, I wrote a blog called Privacy and Humanity on the Brink, looking at many of these AI technologies and questioning whether their wholesale adoption risks dehumanizing us in some ways. My sister, who's a physician, actually challenged me and said, "Well, sometimes AI, particularly in healthcare using applications like what you just described, can actually help rehumanize the system."
By liberating the physician to be able to spend more quality time with their patients, as opposed to all the administrative burdens. So it's a very valid point and one that I think we need to consider when we're considering both the benefits and risks of AI. But as we've discussed, built into those applications and those benefits, we have to address things like bias, safety, patient consent and autonomy.
Especially, transparency and security in the data that is used and shared. I want to just look into the future a little bit and ask you what advancement do you foresee in the next five years, say, of AI and machine learning in healthcare?
DS:
What I'm really excited about in the next five years, is we're going to see a convergence of innovation in a bunch of different fields occur, which will then allow us to responsibly use machine learning and AI technologies to facilitate better care delivery. Our ability to take data to build novel machine learning models that help to solve real patient problems is rapidly improving, so that's exciting.
But what I'm even more excited about to a certain extent, is innovation in the policy space and the social sciences space is also happening. We're seeing how regulatory reform around privacy and patient safety, and data governance and the regulation of when can we use AI and not is also starting to be innovated. In a way that then puts the two together, technology and policy, to then be able to think through how do we actually deploy the technologies?
For a long time, we've been building really cool things that couldn't actually get to the bedside, because we didn't have the policy frameworks around it to enable it in a safe way, and now we do, this convergence is happening. I think in the next five years, you're going to see these technologies actually being turned on.
When you arrive to our emergency department for common conditions, machine learning algorithm may automate ordering a test, and you can get it done that much faster and get to your diagnosis that much faster. I foresee these little areas of automation, whether it's test ordering, prediction, getting to a diagnosis better and in a treatment faster, I think will be game-changing for healthcare. And we'll see them actually start to turn on in the next five years.
When you start to go out 10 years, you start to see a convergence of AI models being embedded into hardware devices that I think will create massive transformation. We'll have to see how we ensure that that transformation maintains patient values, respect privacy and governance along the way.
PK:
As you know, Ontario has just recently tabled Bill 194 that looks to address cybersecurity and AI, and proposes to develop the kinds of policy guardrails and regulatory guardrails that we need. We're just at the beginning of that discussion, and certainly I know our office will be involved in sharing our views.
I hope that you and our listeners will also engage in that very, very important policy debate, so that the governance structures, as you say, evolve alongside the technology, and provide that level of security that patients need to trust the system. I want to ask you one last question if I may, and it's a question I ask many of my guests.
That relates to my office and our strategic priorities. One of them is trust in digital health, where our goal is to promote confidence in the digital healthcare system, by guiding health information custodians to respect privacy and access rights. And also support the pioneering use of personal health information for research, analytics that serves the public good.
What's your advice for my office? How do you think we can help support the responsible use of AI in healthcare, while ensuring privacy is protected?
DS:
I think the advice I would give to your office, is to remember that the policy innovation that's happening is quite literally going to impact the next version of that kiddo who comes through an emergency department. Because if this policy can be generated with speed, with rigor, with quality, and then deployed in a way that truly enables the country to come together to leverage machine learning to improve patient care, it quite literally will save people's lives.
Sometimes as a policymaker, you can feel very, very disconnected from what happens on the ground and the real-world impact. My call-out to your office is to say quite literally, the work you do will save people's lives. Because if you can do that work in a way that allows hospitals like Sick Kids and many other children's hospitals across the country to navigate a complex privacy environment.
To be able to bring machine learning models together, to work collaboratively and to deploy at scale. If your office can help enable part of that, you will contribute to saving lives across the country and it's incredibly powerful.
PK:
Wow, that's certainly a tall order, Devin. Thank you for your advice to our office, and thank you for this great conversation. I've really enjoyed it.
DS:
Thank you for having me. It was so great, and I hope your listeners enjoyed the conversation.
PK:
Well, I'm sure our listeners did find our discussion as inspiring and informative as I did. It's certainly clear that AI has the potential to tackle the healthcare system's most pressing challenges, revolutionizing patient care as we know it. It's also important, as we've discussed, to put in the necessary protections, to ensure that it does so in a way that respects privacy and other human rights that reduces bias, enhances safety and of course, security.
For listeners who want to learn more about AI, digital health and protecting personal health information, I encourage you to visit our website at IPC.on.ca, and you could always call or email our office for assistance and general information about Ontario's access and privacy laws. Thank you all for listening to this episode of Info Matters, and until next time, I'm Patricia Kosseim, Ontario's information and privacy commissioner, and this has been Info Matters.
If you enjoyed the podcast, leave us a rating or review. If there's an access or privacy topic you'd like us to explore on a future episode, we'd love to hear from you. Send us a tweet @IPCinfoprivacy or email us at @email. Thanks for listening and please join us again for more conversations about people, privacy and access to information. If it matters to you, it matters to me.